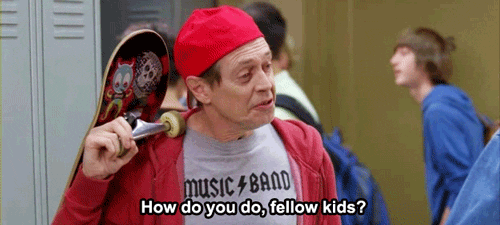
Although the future of AI is a huge topic, many people do not realize the rich and plentiful history behind AI, specifically generative AI. Did you know that generative AI goes back nearly nine decades? Yep! Generative AI had its start in the 1930s. Now, what was created then is nothing like the capabilities today, so don’t be expecting neural networks or anything.
As many people tend to not realize it has been around that long and think it is a brand-new thing, I felt it might be worth exploring the past first so that people can better understand the future coming up.
My focus on this post is around generative Artificial Intelligence (GenAI) and the remarkable evolution and advancement it has made over the past nine decades. It has, and continues, to reshape the landscape of technology, creativity, and human-machine interaction. I will be going through a few different important periods of time to give a chronological account of the history of GenAI. Follow along to learn how over the years generative AI has transitioned from simple rule-based systems to complex neural networks.
First, let’s take a quick introduction into what is generative AI so we can have a baseline to understand the timeline.
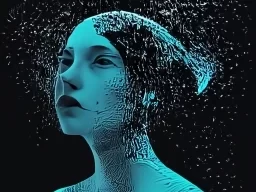
Generative AI (GenAI)
Generative AI is a subfield of artificial intelligence. It focuses on creating machines that exhibit human-like creative behaviors. It refers to a class of machine learning models designed to create new data instances that are similar to existing data. These models are particularly adept at capturing patterns, styles, and structures present in training data and then generating novel content based on those patterns. GenAI encompasses various techniques, including Generative Adversarial Networks (GANs), Variational Autoencoders (VAEs), and Transformer-based models.
Generative Adversarial Networks (GANs)
Variational Autoencoders (VAEs)
These are a pivotal advancement in generative AI, melding autoencoder and probabilistic modeling principles. VAEs are made up by an encoder that converts input data into a latent space, and a decoder that reconstructs data from this space. Notably, VAEs introduce a probabilistic element by mapping input to a probability distribution in the latent space. This probabilistic approach enables diverse data generation and tasks like denoising and anomaly detection, finding applications in diverse fields such as image generation, style transfer, molecular design, and healthcare.
Transformer-based models
Transformer-based models are a transformative leap in generative AI, centered around attention mechanisms for parallelized computation. This architecture excels in tasks like language translation and text generation due to its contextual understanding and long-range dependency handling. Notable examples include BERT and GPT, which have redefined natural language processing by enhancing tasks like sentiment analysis and code generation.
Generative AI holds many very promising applications in the fields of Marketing and IT as well as many other areas such as medicine and healthcare. But, I feel it is best to hold off on looking at the future until we have had the chance to fully explore the past of GenAI.
The History of Generative AI
Below is a comprehensive timeline that chronicles the history of generative AI, exploring its evolution from the early days of computer science to its contemporary state of sophistication. From its inception in the 1930s to its current state in 2023, we will touch and highlight the key developments, influential figures and pivotal moments that have shaped the trajectory of generative AI.
Predecessors and Early Foundations (1933-1955)
- Alan Turing’s “Computable Numbers” (1936) laid the theoretical groundwork for computing and computational creativity.
- Claude Shannon’s “A Mathematical Theory of Communication” (1948) introduced concepts of entropy and information theory, influencing subsequent developments in data generation and compression.
- Early AI pioneers like John McCarthy and Marvin Minsky explored rule-based systems for generating basic patterns and sequences.
The Emergence of Expert Systems (1960s-1970s)
- The development of expert systems marked a shift toward AI applications, with programs like DENDRAL (1965) generating molecular structures and MYCIN (1976) diagnosing infectious diseases
- However, these systems relied heavily on handcrafted rules, limiting their adaptability and generality.
Connectionism and Neural Networks Resurgence (1980s-1990s)
- Connectionist models, including Hopfield networks and backpropagation algorithms, rekindled interest in machine learning and generative models.
- The introduction of Boltzmann machines (1982) and Restricted Boltzmann Machines (RBMs) (1986) paved the way for more efficient training of deep neural networks.
- Early attempts at image generation, such as David Cope’s “Emmy” (1987) and “Experiments in Musical Intelligence” showcased the potential of AI-generated art.
Generative Adversarial Networks (GANs) Revolution (2010s)
- Ian Goodfellow’s introduction of GANs in 2014 marked a groundbreaking milestone in generative AI, enabling the training of generative models through adversarial learning.
- GANs sparked rapid advancements in image synthesis, exemplified by the creation of DCGAN (2015) and subsequent iterations.
- StyleGAN (2019) brought unprecedented realism to AI-generated images by controlling both high-level and low-level features.
Natural Language Processing and Text Generation (2010s)
- OpenAI’s GPT-2 (2019) demonstrated the capabilities of large-scale language models in text generation, raising ethical concerns over potential misuse.
- Fine-tuning GPT-2 led to applications in writing, code generation, and conversation.
Multimodal Generative Models (2020s)
- Recent years have witnessed the emergence of multimodal models, combining text and images for more holistic generative tasks.
- CLIP (2021) enabled cross-modal understanding, facilitating tasks like image captioning and visual question answering.
Current Landscape and Future Directions (2023 and beyond)
- Generative AI continues to evolve rapidly, with ongoing research in novel architectures, improved training techniques, and ethical considerations.
- Future directions include enhanced creativity in AI-generated content, improved generalization, and responsible AI deployment.
The history of generative AI spans nine decades, reflecting the enduring pursuit of mimicking human creativity and ingenuity through machines. From its early beginnings with rule-based systems to the current era of GANs, deep neural networks, and multimodal models, generative AI has made substantial contributions to various domains and will undoubtedly play a pivotal role in shaping the future of technology and human-machine interaction.
With this knowledge, has it changed what you see for the future of AI? Have you been able to get past the buzz and the hype to find the true value? What do you think is the next big thing to come from AI? Is generative AI going to change the market and workplace as much as many are predicting? I guess only time will tell for sure!
Check out this article I made on how leaders should react to generative AI and the questions they should ask or review prior to making decisions.
(Some of this article was generated via ChatGPT…because it’s fun! Can you guess which parts?)